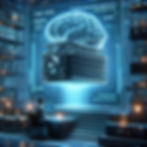
How does QLoRA revolutionize model finetuning and performance?
QLoRA introduces a groundbreaking approach to finetuning large language models, enabling the efficient usage of memory while preserving task performance. This innovative technique allows for the finetuning of a 65B parameter model on a single 48GB GPU, a feat previously thought infeasible. By backpropagating gradients through a frozen, 4-bit quantized pretrained language model into Low Rank Adapters (LoRA), QLoRA achieves remarkable memory savings without compromising on performance.
How does QLoRA impact model performance and efficiency?
QLoRA's impact on model performance and efficiency is profound, as demonstrated by its outperformance of previous openly released models on benchmark tests like Vicuna. The best model family resulting from QLoRA, named Guanaco, achieves an impressive 99.3% of the performance level of ChatGPT while requiring only 24 hours of finetuning on a single GPU. This remarkable performance level, coupled with the efficiency gains in memory usage, signifies a significant advancement in the field of model finetuning.
What makes QLoRA stand out in the field of model finetuning?
QLoRA stands out among other finetuning approaches due to its ability to significantly reduce memory usage while maintaining full 16-bit finetuning task performance. The introduction of novel techniques such as the 4-bit NormalFloat (NF4) data type and double quantization contributes to minimizing the average memory footprint. Additionally, QLoRA leverages paged optimizers to effectively manage memory spikes, further optimizing the finetuning process.
What are the key innovations introduced by QLoRA?
QLoRA introduces several key innovations aimed at optimizing memory usage during the finetuning process. These innovations include the introduction of the 4-bit NormalFloat (NF4) data type, which is information theoretically optimal for normally distributed weights. Furthermore, QLoRA employs double quantization to reduce the memory footprint by quantizing the quantization constants. Additionally, the use of paged optimizers helps manage memory spikes effectively, ensuring smooth and efficient finetuning.
How does QLoRA contribute to advancing chatbot performance?
QLoRA's impact extends beyond model finetuning, as it significantly enhances chatbot performance. By finetuning more than 1,000 models and providing a detailed analysis of instruction following and chatbot performance across various datasets and model types, QLoRA demonstrates its effectiveness in improving chatbot capabilities. Moreover, QLoRA's efficient memory usage enables the finetuning of smaller models, leading to state-of-the-art results in chatbot performance.
What alternatives exist to QLoRA in the field of model finetuning?
While QLoRA represents a significant advancement in model finetuning, several alternative approaches exist in the field. Techniques such as traditional finetuning methods and other memory optimization strategies may offer viable alternatives depending on specific use cases and resource constraints. However, QLoRA's unique combination of memory efficiency and performance preservation sets it apart as a leading solution in the domain of large language model finetuning.
Learn more about AI automation, adoption, and training with ExplainX! Contact us for AI automation, adoption, and training services to empower your organization in leveraging cutting-edge AI technologies.